Data, especially big data, is the new oil in the digital economy; still, harnessing its full potential has tested even the most progressive of organizations. As we navigate through 2024, one persistent challenge that companies face is utilizing data analytics effectively for process improvement. For businesses seeking to streamline their operations and increase their profitability, these challenges can appear as high-tier hurdles. This blog post will unravel those challenges and equip you with an actionable roadmap to overcome them with insights drawn from Big Data. So buckle up because you’re about to learn how to make your data work smarter—not harder—for your business!
The main challenges in using data analytics for process improvement include collecting and managing large amounts of data, ensuring the data is meaningful and real-time, visualizing the data effectively, integrating data from multiple sources, accessing inaccessible data, dealing with poor data quality, facing pressure from management, lacking support and resources, feeling confusion or anxiety around data analytics, dealing with budget constraints, skill shortages, and scaling data analysis. Our website provides a detailed exploration of these challenges and offers potential solutions to overcome them.
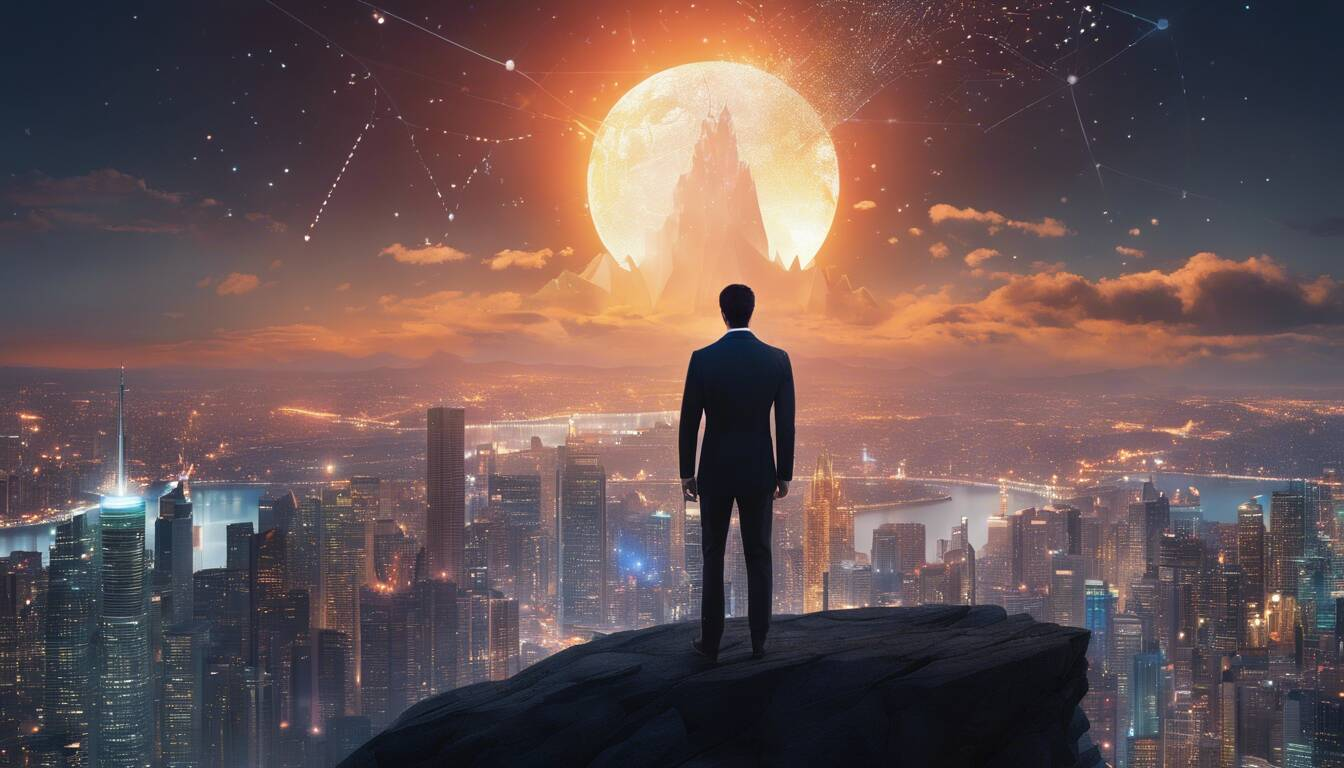
Addressing Data Analytics Challenges for Process Improvement
As businesses seek ways to streamline their operations, it's become apparent that data analytics is a crucial tool for driving process improvements. However, this can be easier said than done, given the hurdles organizations face in implementing big data insights. In this article, we delve into some of these challenges and how businesses can address them.
According to Gartner, 87% of organizations have low business intelligence (BI) and analytics maturity, creating a big obstacle for those wishing to improve their operations through data analysis (2019).
A NewVantage Partners survey (2020) reveals that 77% of businesses report that business adoption of big data and AI initiatives continues to represent a significant challenge.
An Accenture study (2018) indicates that while 79% of enterprise executives agree that companies that do not embrace big data could face extinction, only about one-third have developed a data-driven culture.
Roadblocks in Implementing Data Analytics
Data analytics presents numerous benefits in process improvement, from reducing costs to boosting productivity and customer satisfaction. Still, many roadblocks might prevent an organization from realizing these benefits.
One of the most significant challenges is data integration. Data integration is the process of combining data from multiple sources to provide comprehensive and meaningful insights. Different data sources have diverse formats and structures, adding complexity to integrating datasets effectively. Other difficulties include data security and privacy concerns, exponential growth of data volume and diversity, ensuring data quality and consistency, data compatibility, data security and resilience, and real-time data integration.
Another challenge lies in skilled labor shortages. According to reports by McKinsey & Company, "By 2018, the United States alone could face a shortage of 140,000 to 190,000 people with deep analytical skills as well as 1.5 million managers and analysts with the know-how to use the analysis of big data to make effective decisions." The required knowledge span varies across fields such as statistics, machine learning, and programming languages like Python or R.
Consultants are available to offer solutions on-demand instead of hiring full-time employees for part-time projects; Universities like IBMlearn offer virtual courses on the latest technologies; bootcamps cover full-stack Machine Learning courses with hands-on experience, which helps organizations cross-skill their existing employees
Overcoming such challenges requires a dedicated team-driven culture focused on leveraging technology at decision-making levels. The power of developing diverse teams where staff from different departments join forces to tackle these challenges should be harnessed.
Difficulties in Measuring Process Improvement
In the world of process improvement, measuring the effectiveness of changes made can be challenging. It's crucial to identify the right metrics that accurately depict improved process performance - and what gets measured should align with the objectives and goals set forth. However, many organizations make the mistake of identifying too few or too many metrics without linking them to process improvements.
For example, if a manufacturing company identifies a lack of efficiency in its production line, it may introduce changes that improve workflow, increase productivity, and reduce waste. Yet, measuring solely profits or productivity may not necessarily be sufficient indicators of improvement. Instead, identifying more specific data points, such as cycle time reduction per unit produced, will provide a more complete picture.
Therefore, it is vital to use reliable data to measure progress accurately. This is where leveraging data analytics becomes incredibly useful.
Leveraging Data Analysis for Enhanced Operations
Using big data insights for process improvement is no longer just an option for businesses; it's essential - especially considering that such efforts can lead to cost savings and better decision-making. Not only do these techniques help in understanding existing inefficiencies, but they also provide insights into predicting future issues in various performance areas.
Data analytics helps organizations to identify patterns and trends that are otherwise invisible on spreadsheets or recording sheets. By implementing automated systems that provide real-time feedback, companies can perform statistical analyses that detect potential problems much earlier than traditional methods allow. In turn, this information can help stakeholders make decisions faster and more efficiently.
Think of data analysis as opening a window with a better view of the journey ahead - helping you spot obstacles quicker and navigate through them more effectively.
The goal is to extract meaningful insights from vast amounts of raw data by utilizing cutting-edge technologies like Artificial Intelligence (AI), Machine Learning (ML), Process Mining (PM), and Robotic Process Automation (RPA). These technologies can help businesses evaluate data from diverse sources and make sense of the various aspects of performance metrics, thus making it easier to interpret.
Now that we have established why leveraging data analytics is important for enhanced operations let's dive into specific ways it can identify key performance indicators.
Identifying Key Performance Indicators
When implementing data analytics for process improvement, it's essential to identify the right key performance indicators (KPIs) to track. KPIs serve as measurable values that indicate a company's success in achieving its goals. By identifying the relevant KPIs, organizations can monitor their progress towards specific objectives and make informed decisions.
For instance, a manufacturing firm might set goals to reduce waste by 5% or improve production efficiency by 10%. In these cases, tracking metrics such as waste generated per unit or average cycle time, respectively, could be useful KPIs to gauge progress.
Organizations need to choose KPIs carefully and avoid measuring every available metric. It's crucial to identify a few critical metrics with meaningful impact on business outcomes.
Effective Strategies to Overcome Analytics Obstacles
While data analytics offers a wealth of insights and opportunities for process improvement, there are hurdles organizations must overcome along the way. The following strategies are effective in addressing these common analytics obstacles:
1. Data quality management: Ensuring accurate and consistent data is vital in deriving valuable insights from analytics. Organizations need to establish processes for data governance, including procedures to detect and remove errors or duplicates in data sets.
2. Change management: Adopting new technologies or processes requires careful change management planning. Employees may resist new systems or procedures if they don't understand their benefits and potential impact on daily operations.
3. Skill development: Competent staff capable of conducting analysis and interpreting results is essential for effective data-driven decision-making. Organizations must invest in talent development programs to enhance analytical skills throughout the business functions.
Think of a football team wanting to improve their performance by reviewing footage of their past games - without a good analyst who knows what to look out for, they're likely to miss important observations.
4. Collaboration across departments: Cross-functional collaboration can help organizations identify process improvement opportunities and flush out any inefficiencies across business functions. Interdisciplinary teams working together could uncover fresh insights and tackle various complex challenges.
With these strategies in mind, organizations can overcome analytics obstacles and leverage big data insights to transform challenges into opportunities for process improvement. In the final section, let's delve deeper into how big data insights can precisely do that.
Transforming Challenges into Opportunities with Big Data Insights
As the volume of data continues to grow at unprecedented rates, so do the challenges that come with it. Given that much of this mountain of data is in unstructured form and comes from multiple sources, extracting insights that truly align with business goals is a difficult task. However, such challenges should not be seen as insurmountable, and businesses must adopt a proactive approach to harnessing big data.
First and foremost, businesses need to evaluate their current data strategies. Are they optimized for the current digital environment? Is their underlying infrastructure capable of handling large amounts of both structured and unstructured data? Having answers to these questions go a long way in unlocking the full potential hidden within datasets.
Big data insights allow organizations to improve decision-making processes across different departments. Retailers can use insights to understand consumer behavior better and market trends, while healthcare professionals can create more personalized treatment plans for patients based on their medical history and present state of health.
Moreover, predictive analytics has made it easier for organizations to steer clear of disruptions within supply chains by predicting demand changes and inventory fluctuations. The power that big data insights offer transcends different spheres and can make traditional processes relevant again.
For instance, product quality control has been an age-old process where samples are taken after production, and QA checks are conducted. With big data insights in tow, manufacturers can now monitor production processes in real time by analyzing sensor readings and making adjustments without stopping production altogether. This translates into less downtime, fewer discrepancies, reduced costs, and improved customer satisfaction.
A lot is also happening in stream processing - the ability to analyze incoming data streams in real-time instead of storing them for later analysis has disrupted conventional business practices positively. Analytics firms have witnessed explosive growth in this area recently, with marked adoption rates among financial institutions.
Table 1: Stream Processing Market Size and Growth Projections
Year | Stream Processing Market Size (USD billion) |
---|---|
2020 | 13.5 |
2021 | 16.2 |
2022 | 20.5 |
2023 | 26.6 |
2024 | 34.7 |
In navigating the challenges that come with big data insights, it's important to consider them as surgery; surgical procedures can be complicated but offer lifesaving benefits once completed. Similarly, while navigating the nuances of massive datasets might be daunting, adopting proactive approaches toward overcoming challenges can unlock opportunities for businesses to thrive.
Big data insights offer endless possibilities for businesses but should not be approached carelessly. The allure of limitless data is attractive, but businesses need to ensure they have a robust infrastructure in place and establish well-defined strategies before engaging in collecting and analyzing large amounts of data.